Understanding the Median: Key Insights in Statistics
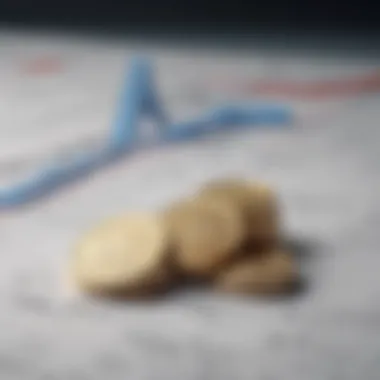
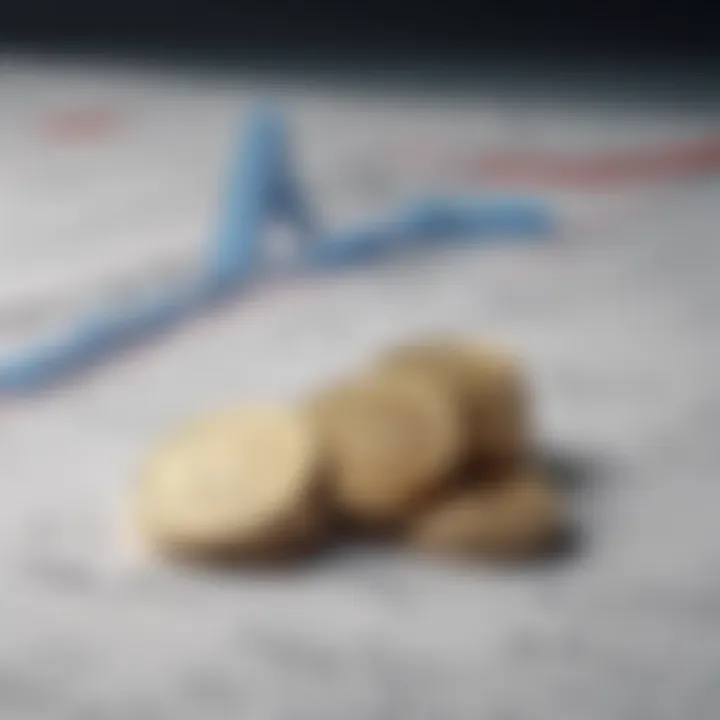
Intro
The median is often what gets lost in the shuffle when talking about statistics. While many people are familiar with the mean, or average, the median offers a different perspective on data. It serves as a powerful measure of central tendency, one that's especially useful when dealing with skewed distributions and outliers. Unpacking the median gives us invaluable tools to better understand data sets, especially when the numbers are tricky and donāt tell an obvious story.
Why Median Matters
Why should anyone care about the median? It's because it reveals the middle ground, the halfway point where half the data lies below and half above. In todayās world, where data is the new oil, grasping the ins and outs of median can build a solid foundation for data interpretation. Whether you're analyzing household incomes or student test scores, the median can provide a clearer insight than the average, especially when faced with extreme values. This brings us to a deeper dive into its calculation and significance.
Investment Dictionaries
Key Financial Terms Explained
In finance and statistics alike, there are terms that often get muddled together, leading to confusion. Hereās a breakdown of those that most directly relate to the median:
- Mean: The sum of all values divided by their count. It's sensitive to extremes, which gives it a tendency to skew the overall perspective of data.
- Mode: The value that appears most frequently. It's useful, but in many cases, it leaves out the nuances we find in the median.
- Quantile: Values that divide the data into equal-sized intervals. The median is the second quartile in this framework, sitting proudly at the 50% mark.
Understanding these terms is vital when navigating through data-heavy discussions. It creates a vocabulary that helps in clear communication, especially when dissecting financial reports and trends.
Understanding Investment Jargon
The financial sector is filled with jargon, and as an investor or an advisor, it's crucial to cut through the noise. Knowing terms like central tendency, distribution, and outlier not only bolsters your statistical vocabulary but also aids in making sense of various reports. Each term plays a role in getting to the heart of your analysis.
For instance, the median shines when examining income distribution not just by highlighting the general trends but by laying bare the true landscape of socio-economic divides. This is where we see the practical implications of median in real-world applications, showing financial advisors a clearer picture when recommending strategies to clients.
"Understanding the median can transform how you interpret investments and financial data; it provides clarity in the chaos of numbers."
Expert Advice
Tailored Investment Strategies
When devising investment strategies, knowing how to interpret medians within data can guide your decisions. Investors should pay close attention to the median income trends when assessing market opportunities. Rather than simply focusing on the average income, examining the median can expose areas where there's potential for growth or indicate risks tied to higher volatility.
Risk Management Tips
Managing risk requires a nuanced approach. The median helps in realizing which data points are outliers that could skew your risk assessment. By factoring in the median, an investor can recognize a normal range, setting benchmarks that are protective in fluctuating markets.
Understanding how the median plays its part in risk evaluation and investment strategy formulation is non-negotiable for anyone in the financial field. This understanding helps you navigate through the financial storm, ensuring you're not swayed by the outliers that can lead to poor judgment.
By grasping these concepts, one can better adjust their approach to investments, ensuring a balanced strategy that recognizes solid data evaluation methods.
Prologue to Statistical Measures
Statistical measures provide essential tools for analyzing data, allowing us to gain insight into complex datasets. Among these measures, the concept of central tendency stands out, serving as a foundation for understanding how data points cluster. By identifying the middle ground within a set of numbers, we can summarize large amounts of data with significant efficiency. This article will explore the median, a key measure of central tendency, and its crucial role in statistical analysis.
Overview of Central Tendency
Central tendency refers to a central or typical value for a probability distribution. It gives us a concise summary that represents a whole dataset. The three primary measures of central tendency are mean, median, and mode.
- Mean: The arithmetic average of a set of values. While it provides a general sense, it can easily be skewed by extreme values.
- Median: The middle value that separates the higher half from the lower half of a data set. It is often a better measure when dealing with skewed distributions.
- Mode: The most frequently occurring value in a dataset.
Understanding these measures is crucial for anyone dealing with data, whether in finance, education, or research. They help paint a clearer picture of what the data means in practical terms. By focusing on where data tends to concentrate, stakeholders can make informed decisions.
Why Median Matters
The median holds a special place among the measures of central tendency, particularly for its resilience against outliers. In a world where extremes can distort perception, having a measure that reliably reflects typical values is invaluable. For example, consider a dataset representing household incomes in a region. If one household earns ten million while others earn far less, the mean income would be driven up skewing the overall reflection of income distribution. In contrast, the median would remain more stable, offering a truer representation of the typical household's economic situation.
In many statistical analyses, such as survey data interpretation or real estate valuation, the median often provides a clearer picture than the mean. Thus, understanding how to calculate and interpret the median will benefit investors, educators, analysts, and financial advisors alike. With the growing emphasis on data-driven decision-making across industries, familiarity with the median undoubtedly enhances one's ability to draw actionable insights from the numbers at hand.
"The median is often the unsung hero in data analysis, quietly providing clarity amid chaos."
In summary, as we delve deeper into the median within the context of statistics, we will explore its definition, calculation, and application in various fields. This exploration not only enhances our statistical toolkit but also enriches our understanding of the data landscape.
Defining the Median
The concept of the median stands as a cornerstone in the field of statistics. It's not merely a calculation but a critical insight into understanding data distributions. The median helps reveal the center of a data set, offering clarity, especially when faced with varying datasets that don't always follow typical distributions. Importantly, the median is especially beneficial in skewed distributions where outliers might distort the representation given by other central tendencies, such as mean. Basic understanding will help investors, financial analysts, and educators navigate complex data with greater ease.
Basic Definition
At its core, the median represents the middle value of a dataset when sorted in increasing order. If you line up all the numbers from smallest to largest, the median is the one sitting right in the middle. For example, consider the dataset: 3, 7, 9, 15, and 20. When arranged, the number 9 is smack in the middle ā thatās the median. Conversely, for a dataset comprising an even number of observations, say 4, 8, 15, and 16, the median would be the average of the two central values (8 and 15), which calculates to 11. This property allows the median to serve as a reliable indicator of central tendency, often reflecting the true central point of data better than the mean, particularly in skewed distributions.
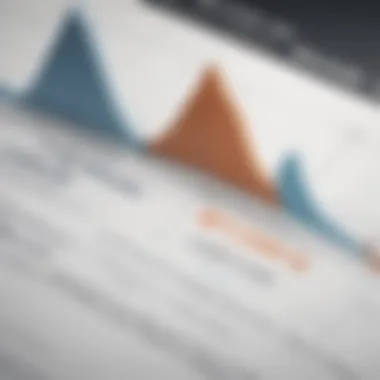
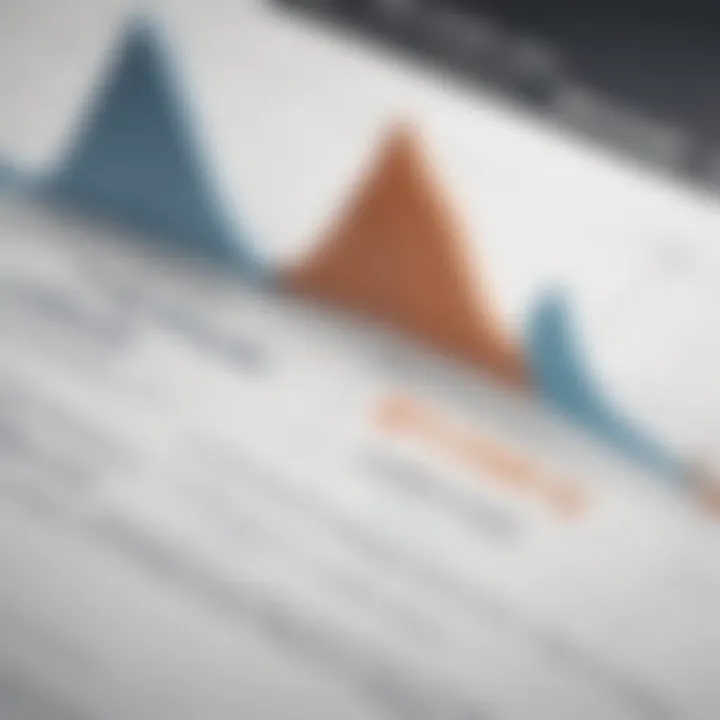
Mathematical Representation
In mathematical terms, the median can be represented in a straightforward manner:
- For an odd number of data points, the median is defined as:[
extMedian = x_(n+1)/2
]where ( n ) is the number of observations. - For an even number of data points, the median is calculated as:[
]This ensures that the median properly reflects the central point by adjusting for even sets. It distinctly highlights the medianās capability to handle datasets with fewer complications compared to the mean, making it a favored choice for analysts working with financial data, survey results, or any field where data integrity is vital.
"The beauty of the median lies in its resilience against extremes, providing a clearer picture of typical values in data analysis."
Understanding these definitions and representations sets the stage for broader discussions on calculating the median and applying it in real-world scenarios, ensuring that the readers truly grasp this valuable measure.
Calculating the Median
Calculating the median is a pivotal aspect of understanding statistical measures. The median serves as a reliable descriptor of central tendency, offering an insight into the distribution of data. Unlike the mean, which can be greatly affected by extreme values or outliers, the median remains steadfast, providing a clearer picture of the data's center. This is especially crucial in fields like finance and education, where accurate data interpretation can inform better decision-making.
Here, we will break down the calculation methods for different types of data sets while considering the advantages and potential pitfalls inherent in each approach.
Median of Odd-numbered Data Sets
When dealing with an odd-numbered set of values, finding the median becomes straightforward. Simply arrange the data points in ascending order and pinpoint the middle value. This method allows for a concise determination of the median, as there will be one value directly at the center.
For instance, consider the following set of values: 4, 8, 15, 16, 23. The first step is to arrange them: 4, 8, 15, 16, 23. The middle value, in this case, is 15, making it the median.
To put it simply, the formula used can be described as follows:
- Arrange data points in ascending order.
- Locate the middle point:
- If the total count of data points is odd, the formula applied is: Median = Data a[(n + 1) / 2]
This approach is beneficial because it exclusively focuses on the middle value, ensuring that all surrounding values do not skew the median.
Median of Even-numbered Data Sets
Unlike odd-numbered data sets, even-numbered collections can pose a slight challenge when it comes to median calculation. With pairs of middle values rather than a single center point, calculating the median requires a bit more work.
Letās say we have a data set: 3, 7, 9, 10, 12, 14. After arranging it in order, we see it remains as is since it's already organized: 3, 7, 9, 10, 12, 14. The two middle numbers here are 9 and 10. Since there is no single value at the center, the median is calculated by averaging these two middle numbers:
Median = ( + ) / = 9.
The steps for finding the median in even-numbered data sets are as follows:
- Arrange the values in ascending order.
- Identify the two middle values and calculate the average:
- If the total count of data points is even, use the formula: Median = (Data[(n/2)] + Data[(n/2) + 1]) / 2
This method ensures that the median reflects the central tendency of the data set even when there isn't a clear middle point.
Techniques for Unsorted Data
In the real world, we often encounter data that is not neatly sorted out. In such cases, you may need to implement specific techniques to find the median accurately. Hereās how you can navigate this:
- Sort the data: The first step, regardless of the initial state, is to arrange the values in ascending order. Even if this requires additional time, it's critical for accurate median computation.
- Identify the count of values: Determine whether your total number of values is odd or even.
- Calculate the median: Use the previously mentioned formulas for odd or even datasets after you've sorted the data.
Using software or statistical tools can also ease this process significantly. Most statistical software quickly compute the median for unsorted datasets. However, understanding the mechanics enhances your analytical skills.
Median vs. Other Measures of Central Tendency
When navigating the realm of statistics, the discussion of central tendency measures feels akin to comparing apples to oranges. Each measureāmedian, mean, and modeācarries weight and relevance, but the context dictates which one shines brightest in a given circumstance. Here, we can explore the nuances that separate the median from its counterparts.
Comparison with Mean
To understand the median's place within central tendency, it's crucial to examine how it stands against the mean, which is perhaps the most well-known measure. The mean, often referred to as the average, is calculated by summing all the values in a data set and dividing by the total number of observations. This seemingly straightforward method is often hampered by outliers; a single extreme value can significantly skew the result. For instance, consider the income of five individuals: $25,000, $30,000, $35,000, $40,000, and $1,000,000. The mean income here jumps to $226,000, which hardly reflects the typical earnings of the majority in this group.
In contrast, the medianāthe middle value when all observations are arranged in orderāprovides a clearer picture of the central tendency, especially in skewed distributions. The median income would be $35,000 in this scenario. By focusing solely on the middle value, the median avoids being thrown off by that millionaire in the mix, making it often more representative of the typical case in situations rife with outliers.
"In statistics, while the mean might be the star of the show, the median is the unsung hero, revealing insights without the noise of extreme values."
Comparison with Mode
Next, letās turn our gaze towards the mode, the value that appears most frequently in a data set. The mode is particularly useful for categorical data, where you might want to identify the most common category or item. However, itās worth noting that a data set can have more than one mode, or even none at all. This characteristic can lead to ambiguity when trying to define the central value of a data set.
While the mode can offer insights into the popularity of a particular outcome, its limitations become clear when one desires to gauge elevation or balance in a numerical dataset. Consider a simple example: the numbers 2, 4, 4, 6, and 8. In this case, 4 is the mode, but it doesnāt tell you much about the overall distribution of numbers. The median, on the other hand, would again be 4 in this instance but provides context alongside the mean, in this case 4.8, allowing for a more rounded understanding of where the bulk of data lies.
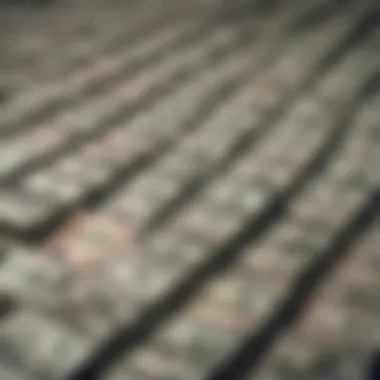
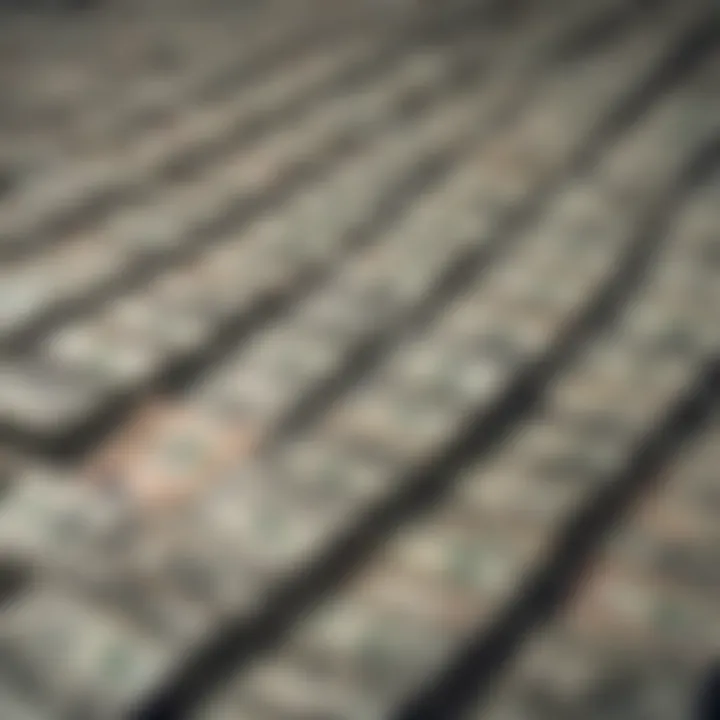
In summary, the trio of median, mean, and mode each serve distinct purposes, with the median carving a niche particularly when data distributions are uneven. When figuring out the measure to use, itās essential to consider the nature of the data at hand. The decision can lead to tackling more accurate analyses and meaningful interpretations, and more importantly, informed decision-making.
Applications of the Median
Understanding the median's applications offers a window into its practical relevance. The median serves as a compass for navigating through myriad statistical waters, guiding insights in ways that raw data often fail to do. Its impact is particularly pronounced in fields such as economics, real estate, and market research, where decision-making hinges on understanding distributions effectively. Below, we explore three vital areas where the median holds immense significance.
Income Distribution Analysis
When assessing income distribution, merely looking at averages can lead to misconceptions. Consider this: a handful of billionaires can skew average income figures for an entire population. Here, the median shines brightly. It offers a more balanced perspective by highlighting the middle point, dividing the income levels exactly in half.
For instance, in a community where eight families earn an annual income of $40,000 and two families earn $1,000,000, the average income might present a misleadingly rosy picture of wealth distribution. In contrast, the median income would reveal that half the families earn below $40,000. This can inform policy decisions and economic measures aimed at addressing income inequality.
Real Estate Valuation
The real estate market is notorious for its volatility, where property values can fluctuate dramatically based on various factors. Here, the median sale price is a vital tool for both buyers and sellers. It eliminates the noise created by extreme highs and lows.
Think about a neighborhood where a few properties are sold for exorbitant prices, perhaps due to unique attributes or historical significance. If one home sells for $1.5 million while others go for $400,000, the average sale price would be skewed. The median sale price, however, would offer a clearer picture of what most buyers are willing to pay. Thus, the median can empower both buyers and sellers in negotiating transactions, evaluating options, or even planning renovations.
Survey Data Interpretation
Surveys are often the backbone of market research and policy formulation. However, analyzing survey results can be tricky when respondentsā answers vary widely. Hereās where the median steps into the spotlight again. It provides a summary statistic that can represent a typical response without getting derailed by extremes.
For instance, if a survey about customer satisfaction scores yields results like: 1, 2, 3, 4, 5, 10, the mean comes out as 4.14, which doesnāt reflect the experience of most respondents. On the other hand, the median is 4, accurately reflecting the central tendency of satisfaction levels.
The take-home from these applications is clear: the median is not just a theoretical concept; it's a critical instrument for making sense of data in real-world scenarios. It encapsulates trends and highlights concerns that might be overlooked if one were to rely solely on averages.
Advantages of Using the Median
When it comes to analyzing data, the median offers some unique perks that canāt be overlooked. This measure makes waves in various fields, from finance to education. The importance of the median can be boiled down to a few fundamental elements that make it a stand-out choice over other statistics.
Robustness to Outliers
One of the strongest attributes of the median is its ability to withstand the onslaught of outliersāthose pesky data points that can skew results in a heartbeat. You see, when you have a bunch of numbers, letās say, incomes of a small business team, an extremely high or low earner can throw the average (mean) way off balance. Imagine a small company where most employees earn between $40,000 and $60,000, but the CEO rakes in $300,000. The mean salary would jump significantly higher than the majority of the teamās actual earnings, giving an inaccurate picture of what most earners pull in. The median, however, sits just comfortably at the halfway mark, representing the middle without being affected by those outliers, thus offering a clearer snapshot of whatās really going on.
Thereās no need for complex calculations; itās simply the middle value that shields itself from the chaos of extreme deviations, making it a better choice when accuracy is paramount.
Clear Interpretation
Another advantage lies in the straightforwardness of the median's interpretation. Anyone can grasp the concept of the middle value. When youāre presenting data, especially in a meeting filled with a mixed bag of folks from different backgrounds, steering clear of jargon becomes crucial. Saying, "The median income for our team is $55,000" is to the point, easy to digest, and leaves little room for misinterpretation.
Unlike the mean, which often needs some added context, especially when outliers are present, the median is just what it isāa benchmark. This ease of understanding allows stakeholders to make quick, informed decisions based on reliable insights.
"In the world of data analysis, clarity is king. The median shines brighter than most, offering reliability in interpretation that can guide strategic choices."
In essence, understanding where your data tends to sit allows for better planning and resource allocation. Whether for personal finance, like analyzing expenses, or business decisions, a clear picture of central tendency is key.
By leveraging these advantages, the median isnāt just a statisticāitās a trusty compass guiding informed decisions.
Limitations of the Median
While the median can be a powerful tool in statistics, it is far from perfect. Understanding the limitations of the median is crucial for anyone working with data, be it investors, analysts, or educators. These limitations underscore the need for careful interpretation and context when discussing central tendency measures.
Loss of Data Insights
One of the significant drawbacks of using the median is that it can obscure valuable information contained within the dataset. For example, suppose you have a set of annual incomes for a group of individuals:
- $30,000
- $35,000
- $45,000
- $50,000
- $300,000
Here the median income would be $50,000; however, this figure fails to represent the reality of income distribution effectively. The presence of the individual earning $300,000 skews perceptions of income levels, yet the median doesn't reflect this. This āhidingā of data insights can lead to half-baked conclusions and decisions.
When decision-makers rely solely on the median without analyzing other aspects of the dataset, they risk missing out on crucial context that can inform their strategies. Here,ā quantitative analysis should be a blend of main statistics and an understanding of the associated data shape.
Limited Applicability in Distributions
Not every distribution is ideal for median analysis. In certain scenarios, particularly with highly skewed data distributions, the median's usefulness can diminish. Consider, for example, a dataset where the most common values are clustered at one end, and a few extreme values lie at the other:
- Values: 10, 12, 13, 14, 15, 20, 100
This set has a median of 14, yet the mean would be significantly higher due to the one extreme outlier of 100. In this case, it would be misleading to rely solely on the median when attempting to understand the actual values within a dataset.
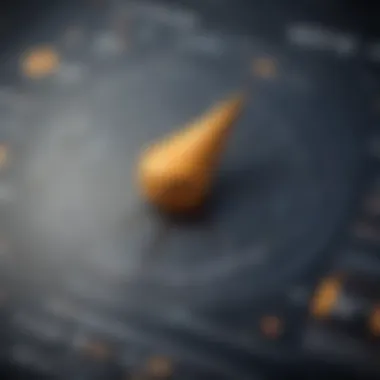
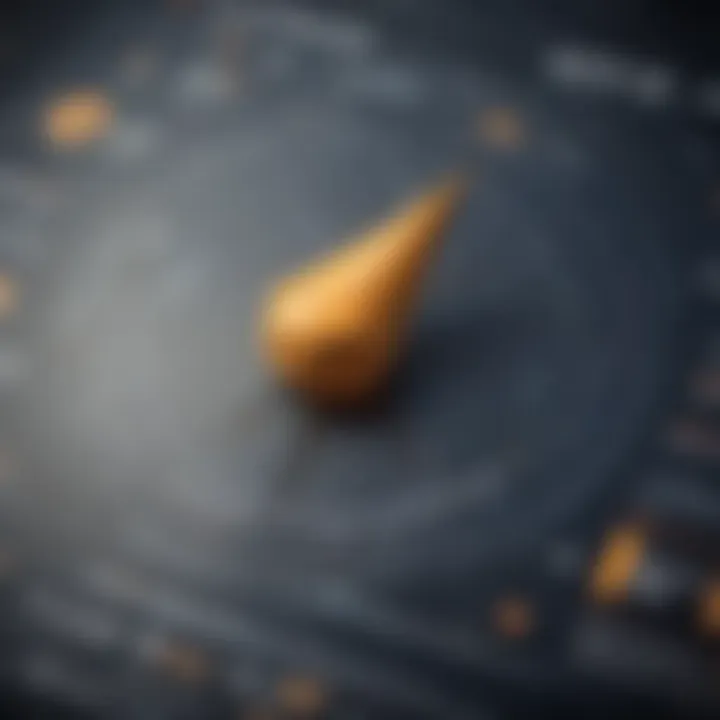
Moreover, in normally distributed data, the mean, median, and mode align neatly, confirming each otherās conclusions. However, in skewed distributions, they can diverge, leading to confusion regarding a dataset's central tendency. Relying too heavily on the median in these instances might cause a lack of insight into discrepancies or the need for further investigation.
In summary, while the median is a valuable statistic, its limitations must be acknowledged to ensure comprehensive data analysis. When making decisions based on statistical data, always consider the context, explore varying measures, and remember that every statistic can tell a different story when viewed through the right lens.
The Role of Median in Decision Making
In the realm of decision-making, various statistical measures hold invaluable weight. Among them, the median stands out for its distinct properties and practical applications. This section explores how the median serves as a crucial tool in steering choices that impact both personal finances and business analytics. Its ability to present a more reliable picture of central tendency, especially in skewed distributions, makes it imperative for informed and strategic decision-making.
In Personal Finance
When it comes to managing oneās finances, understanding the implications of median values can make a significant difference. Take, for example, household income statistics. If one were to glance at averages, those figures can often be distorted by high earners. In contrast, the median income presents a more accurate reflection of what a typical household earns. Hereās how this plays out:
- Budgeting: When setting up a budget, knowing the median income helps individuals align their expenditures realistically. It aids in determining how much one can afford to spend on housing, food, and leisure without breaking the bank.
- Loan Applications: Financial institutions often look at median income levels when assessing loan applications. An applicant's acceptance could greatly hinge on how their income stacks against the median, ensuring that lending practices are in line with market standards.
- Investment Decisions: Investors can harness median data to gauge the financial health of a market or sector. For instance, knowing the median price of properties in a certain area can inform decisions on whether to buy or sell, avoiding overvaluation or undervaluation of assets.
The median, therefore, empowers individuals to make decisions based on reality rather than illusions created by averages that might skew towards extremes.
In Business Analytics
In the realm of business, the median serves as an invaluable asset in data analysis, facilitating insights that guide strategic pathways. Here's how this metric enhances decision-making in various business scenarios:
- Performance Metrics: Companies often use median figures to assess employee performance. For example, if a sales team has variances in earnings, evaluating the median can shine a light on the typical sales figure, thus setting more relatable performance benchmarks.
- Customer Feedback Analysis: In survey data, the median can convey the central sentiment among customers. A business might ask their clients to rate satisfaction on a scale from 1 to 10. If the mean score is boosted by a few enthusiastic outliers, the median score will hone in on the experience of the average client. This understanding can direct enhancements in services or products.
- Market Analysis: When exploring client demographics, knowing the median age or income of customers helps businesses tailor their marketing strategies to better attract and serve their audience.
In sum, whether navigating through personal finances or steering the course of a business, the median reinforces clarity in decision-making. Its robustness against outliers and its ability to simplify data interpretations not only enriches insights but also guides strategic initiatives that align with real-world scenarios.
Case Study: Median in Action
To appreciate the true power of the median in statistics, case studies serve as real-world showcases of its applications. This section illuminates the critical role that the median plays in understanding data sets, especially in scenarios where other measures might be misleading. It's particularly useful because it maintains its integrity despite the chaos of outliers, providing a clearer view of the underlying trends. Let's delve into two specific examples of its application: income data from a particular region and customer satisfaction surveys.
Income Data from a Specific Region
Consider a study that analyzes annual incomes in a particular city. Letās say the incomes of ten residents are as follows: 30,000; 28,000; 25,000; 100,000; 35,000; 60,000; 45,000; 80,000; 75,000; and 200,000. At a glance, the average (mean) income growth of this group would be skewed by that one resident earning 200,000, potentially painting an inaccurately rosy picture.
To calculate the median:
- Sort the data in ascending order: 25,000, 28,000, 30,000, 35,000, 45,000, 60,000, 75,000, 80,000, 100,000, 200,000
- Identify the middle value: Since there are ten values (an even number), average the fifth and sixth values.
- Median income: (45,000 + 60,000) / 2 = 52,500.
Here, the median income sits at a more informative 52,500, reflecting the income distribution more accurately. This figure allows policymakers and financial analysts to comprehend the economic landscape without the distortion introduced by that exceedingly high income. Investors can make more grounded decisions when they see this median instead of relying solely on the mean.
Customer Satisfaction Surveys
Turning to another practical example, let's analyze how the median figures into customer satisfaction data collection. Imagine a survey distributed to a specific product's users. The ratings given on a scale of 1 to 10 by a group of customers are as follows: 1, 2, 10, 2, 4, 5, 9, 3, 4, and 8. Here, you can see a wide variety of responses, from very dissatisfied to highly satisfied.
The median rating calculation unfolds like this:
- Organize the ratings: 1, 2, 2, 3, 4, 4, 5, 8, 9, 10
- Locate the median: There are ten ratings (an even count), so average the fifth and sixth ratings.
- Median satisfaction rating: (4 + 4) / 2 = 4.
In this case, the median score of 4 presents a realistic picture of customer perception. While the mean might also be around this level, the median firmly asserts that half the respondents rated it lower than 4 while half rated it higher, signifying a balanced representation of responses.
Without a doubt, the median assists in making sense of complex data sets in these scenarios. Collectively, these case studies underscore how leveraging the median can enhance understanding in diverse areas such as income distribution and customer satisfaction. By focusing on median statistics, investors, business strategists, and decision-makers can navigate the turbulent waters of data interpretation with greater clarity.
Ending and Future Considerations
The conclusion of this article is not just a wrap-up; itās an opportunity to reflect on the role the median plays in the grand tapestry of statistics. As we've explored, the median stands as a distinguished measure of central tendency, shining in its resilience against outliers and its ability to present a clearer picture in certain data distributions. In the world of statistics, where raw data can be overwhelming, having a robust and easily interpretable tool like the median is immensely beneficial. It allows investors, financial advisors, analysts, and educators to glean insights that could influence important financial decisions.
Key benefits of understanding the median include:
- Robust Analysis: The median provides a more stable central point in skewed distributions, allowing for more reliable insights.
- Enhanced Decision-Making: For businesses, using median values can pivot financial strategies, focusing on what truly matters without the noise created by outliers.
- Educational Value: Encouraging students to grasp median's workings promotes critical thinking around data interpretation, which is essential in today's data-driven era.
In considering the future, the significance of the median is likely to grow even more. With the continuous influx of data and the increasingly complex phenomena being studied, techniques will evolve to better calculate and apply medians in ways we might not yet envision. As machine learning and artificial intelligence continue to reshape data analysis, understanding the fundamental statisticsālike the medianāwill remain essential.
"The true essence of data wonāt just be in what it shows, but in what you learn from it."
Grasping how to utilize the median effectively can serve as a cornerstone for making informed predictions and decisions moving forward. Whether in finance, healthcare, or social sciences, the medianās applications are vast, underscoring its timeless relevance in statistical discourse.
Summary of Key Insights
- Resilience Against Outliers: The median simplifies data analysis by remaining unaffected by extreme values, thus providing a more accurate representation of central tendency in varied datasets.
- Versatility Across Fields: Whether itās analyzing incomes or survey results, the median finds relevance across numerous domains, emphasizing its wide applicability.
- Guiding Decision-Making: From strategizing in boardrooms to teaching in classrooms, the median delivers critical insights that shape decision-making processes.
The Evolving Role of Median in Statistics
As we continue to collect and analyze unprecedented amounts of data in various sectors, the importance of the median is becoming clearer. The rise of big data has put the spotlight on how statistical measures adapt to provide clarity in complex scenarios.
In the near future, the use and interpretation of the median may evolve in several ways:
- Integration with Advanced Algorithms: As artificial intelligence and machine learning methods become commonplace, training these systems to recognize and utilize the median effectively could improve predictive analytics.
- Interactive Data Visualization: The adoption of median in data presentation tools might evolve, using real-time visualization to represent data trends and patterns effectively.
- Cross-Disciplinary Applications: Fields like psychology and health sciences, which often deal with ordinal data, may increasingly leverage the median to interpret results, enhancing interdisciplinary collaboration and insights.
In summary, the median's role is not static; it is a dynamic element of statistical analysis that continues to adapt to our needs and the nature of the data itself. As we navigate through this evolving landscape, keeping a pulse on the developments surrounding the median will enhance our capacity to draw meaningful insights from data, ensuring its place as a critical component in the statistics toolkit.