A Comprehensive Guide to Understanding P-Values in Statistics
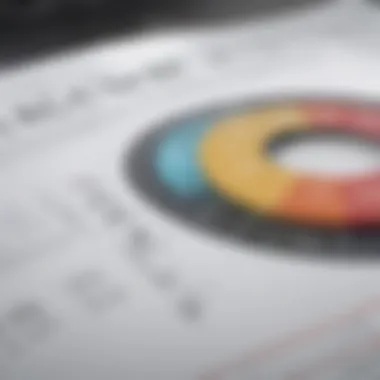
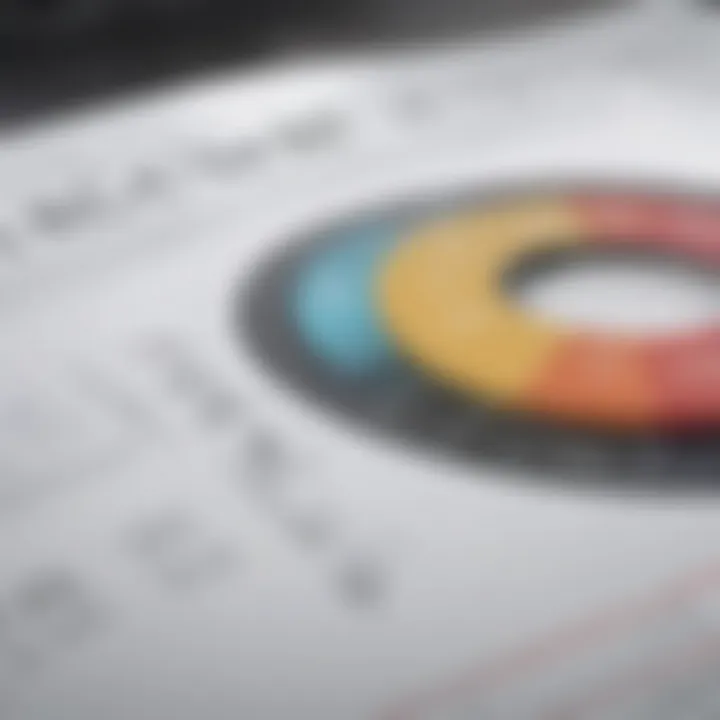
Intro
In the intricate world of statistics, the p-value serves as a crucial benchmark for assessing the validity of hypotheses. It encapsulates the probability of observing results as extreme as those obtained, under the premise that the null hypothesis holds true. Understanding the nuances of the p-value is not only pivotal for researchers but also for anyone engaged in fields requiring quantitative analysis, be it finance, healthcare, or social sciences.
The discussion around p-values often revolves around their role in hypothesis testing. When researchers craft a new study, they typically start with a null hypothesis—essentially a statement of no effect or no difference. The p-value helps them quantify the likelihood of seeing their data if that null hypothesis is correct. This brings about a tension that is fundamental in statistical thinking: how much weight should we assign to these probabilities in the face of uncertainties?
Over the years, the p-value has gained notoriety, often being misinterpreted or misapplied. Thus, there is a pressing need to unravel its complexities and clarify its practical implications. By doing so, we empower not just statisticians but also investors, financial advisors, and anyone involved in decision-making processes based on data.
This article aims to dissect the p-value, moving beyond its mere definition. We will explore its calculation, applications, and common pitfalls, while addressing misconceptions that have pervaded the literature and practice. Emphasizing its relevance across various disciplines, this narrative seeks to enhance comprehension and promote a more nuanced understanding of statistical evidence.
Defining the P-Value
In the realm of statistics, the p-value stands as a cornerstone for interpreting data. This article intends to shed light on its significance while unpacking its nuances in various applications. Understanding what the p-value truly represents is critical for anyone engaging in statistical analysis, whether in finance, education, medical research, or social sciences. Few concepts are as crucial as the p-value in hypothesis testing, making it imperative to grasp how it influences decision-making processes.
Basic Concept
The fundamental notion behind the p-value is straightforward yet profound. It quantifies the probability of observing results, or something more extreme, if the null hypothesis were true. In simpler terms, a low p-value indicates that the observed data is unlikely under the assumption of the null hypothesis. Picture this: if you're flipping a coin, the p-value tells you how reasonable it is to get a series of heads in a row based on the assumption that the coin is fair. If you get heads five times in a row, the corresponding p-value might suggest that such an outcome is rare, prompting you to reconsider whether the coin is indeed unbiased.
Historical Context
Diving into history, the concept of the p-value emerged from the work of statistical figures like Ronald A. Fisher in the early 20th century. Fisher introduced this idea in a 1925 publication, where he emphasized its utility in agricultural experiments. This led to a gradual acceptance of the p-value model in statistical practices. Yet, not everyone jumped on board immediately, and debates persisted over the years regarding its interpretation and application. The p-value ignited discussions around proper statistical methodology, sometimes straying into hotly contested territories, such as what constitutes significant evidence against the null hypothesis.
Mathematical Interpretation
Mathematically, the p-value is often expressed as follows:
[ P = P(X \geq x | H_0) ]\
where X represents the test statistic calculated from the sample data and H_0 indicates the null hypothesis being tested. At its core, this equation illustrates how likely our observed data (or worse) would occur if the null hypothesis were accurate. For stakeholders, investors, and analysts, understanding this equation coupled with its implications facilitates insightful data-driven decision making. Grasping these mathematical mechanics fosters deeper comprehension of the p-value beyond surface-level interpretations.
"The p-value embodies a delicate balance between evidence and probability, emphasizing the significance of statistical reasoning."
This foundational understanding sets the stage for appreciating the role of the p-value in hypothesis testing, where it becomes central to discerning what is statistically significant and influencing subsequent conclusions drawn from data.
The Role of P-Value in Hypothesis Testing
The p-value is a cornerstone in the realm of hypothesis testing. It serves as a numerical representation of the probability of obtaining test results at least as extreme as the observed results, assuming that the null hypothesis is true. This little number packs a big punch, informing researchers whether their findings reflect a genuine effect or if they are merely a product of chance.
Null and Alternative Hypotheses
Before diving into the p-value, it’s critical to understand the framework of hypothesis testing. Every hypothesis test starts with two competing hypotheses:
- Null Hypothesis (H0): This is a statement of no effect or no difference. For example, if a new medication is being tested, the null hypothesis would state that there is no difference in effectiveness compared to a placebo.
- Alternative Hypothesis ( or Ha): This represents what the researcher aims to prove. Using the medication example, the alternative hypothesis posits that the medication does have a significant effect.
Understanding the distinction between these hypotheses helps in interpreting the p-value. A low p-value indicates that the observed data is unlikely if the null hypothesis is true, leading researchers to consider rejecting H0 in favor of . Conversely, a high p-value suggests that the data can comfortably coexist with the null hypothesis, indicating no strong evidence against it.
Significance Levels
The significance level, denoted as alpha (α), is another vital element in hypothesis testing. Traditionally set at 0.05—though it can vary depending on the field—this threshold denotes the probability of committing a Type I error, which occurs when the null hypothesis is incorrectly rejected.
- If the p-value is less than α, researchers reject the null hypothesis, deeming the results statistically significant.
- If the p-value is greater than α, they do not reject the null hypothesis, meaning the evidence is insufficient to support the alternative hypothesis.
This clear-cut approach provides a framework that researchers can follow, but it is also crucial to understand that significance levels can be arbitrary. Hence,
Choosing a significance level requires careful consideration of the implications of potential errors.
Interpreting P-Values
Interpreting p-values isn't just about applying a straightforward rule; it involves a nuanced understanding of the context. For instance, a p-value of 0.03 indicates a 3% probability that the observed data could arise under the null hypothesis. This offers low odds that the results are due to chance.
However, it’s essential to recognize that a low p-value does not imply a greater effect size or practical significance. An effect with a p-value of 0.01 may not hold more real-world relevance than one with a p-value of 0.049. Therefore, researchers should not solely focus on p-values but also consider the size and direction of their effects.
Moreover, p-values operate on a continuum. A p-value of 0.2, while statistically non-significant, can still imply meaningful trends that may warrant further investigation. Therefore, interpreting these values responsibly plays a significant role in scientific communication and data-driven decision-making. In sum, researchers must wield p-values without becoming overly reliant on them, remembering that they serve as a tool rather than an absolute verdict.
By grasping the role of p-values in hypothesis testing, researchers can more effectively draw conclusions from their data, steering clear of pitfalls and embracing a deeper understanding of statistical significance.
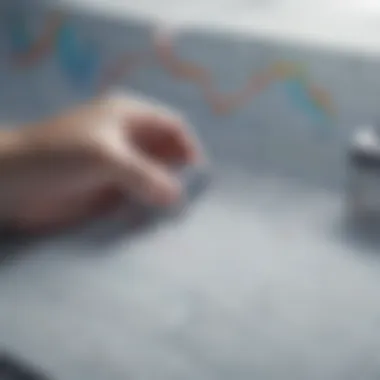
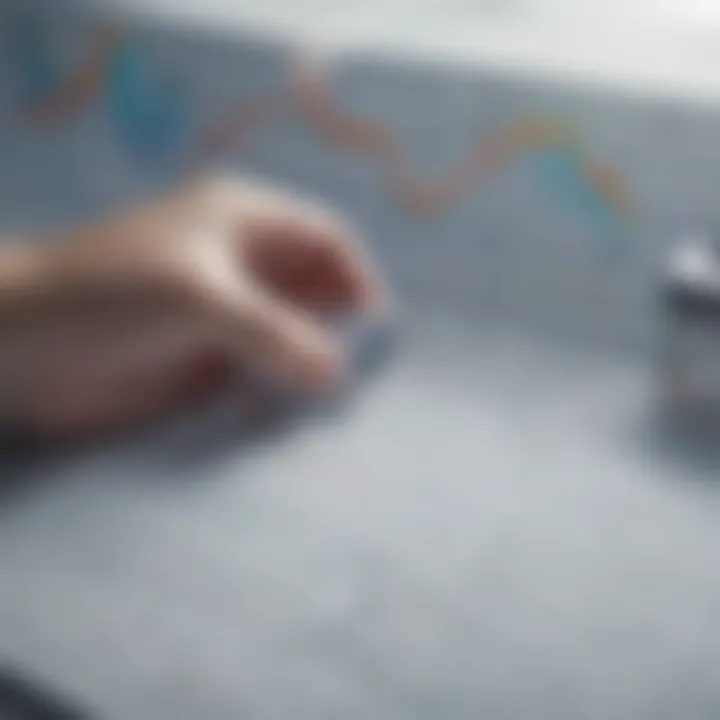
Calculating the P-Value
Understanding how to calculate the p-value is pivotal in statistical analysis. It quantifies the evidence against a null hypothesis, providing clarity when making decisions based on data. Accurate p-value calculation contributes to effective hypothesis testing and drives informed conclusions in various fields such as medicine, social science, and market analysis. The methods can vary depending on the type of data and the hypotheses involved, making it crucial to choose the appropriate method for accurate results, leading to better insights and understanding.
Common Methods
There are several common methods to calculate the p-value, each tailored for specific data types and scenarios. These are usually classified by the statistical tests used. Here are a few prominent ones:
- t-Tests: Used when comparing the means of two groups to see if they are significantly different from each other. A one-sample t-test or two-sample t-test can yield the p-value effectively.
- Chi-Squared Tests: Primarily employed for categorical data to determine if there's a significant association between two variables.
- ANOVA (Analysis of Variance): Useful when comparing means across three or more groups to assess if at least one group mean differs substantially.
- Regression Analysis: Involves estimating the relationships among variables and often includes calculating a p-value for each coefficient to assess its statistical significance.
Each of these methods has its own assumptions and conditions that must be met for the p-values to be reliable. Taking the time to choose the right approach ensures the findings are grounded in solid statistical reasoning.
Software Tools for Calculation
The advent of technology has streamlined the calculation of p-values, making it user-friendly through various software tools. Not only do these tools save time, but they also minimize human error, thus promoting accuracy. Some widely used software include:
- R: A powerful free tool for statistical computing that allows users to perform complex analyses, including custom p-value calculations.
- Python (SciPy): This programming language library offers functions for a variety of statistical tests, which automatically compute p-values.
- SPSS: A comprehensive software for statistical analysis, providing an intuitive interface for performing hypothesis tests and easily extracting p-values.
- Excel: While not a dedicated statistical tool, with the right formulas, Excel can compute p-values for various tests, making it accessible for many users.
Leveraging these tools enhances the reliability of the calculations and plays a significant role in the verification of research findings.
Factors Influencing P-Value
Several factors can influence the p-value, often affecting the interpretation of results.
- Sample Size: Larger samples tend to produce more reliable estimates, which can lead to smaller p-values due to increased statistical power.
- Effect Size: This measures the magnitude of the difference or relationship and can impact the p-value. A larger effect size typically correlates with a smaller p-value.
- Variability in Data: More variability tends to obscure the relationships, which might lead to larger p-values.
- Choice of Test: Different statistical tests have different sensitivities to data, informing how the p-value is derived.
It is critical to understand these factors to avoid misinterpretation and to grasp the context surrounding the p-value, ensuring ethical data analysis practices.
Applications of P-Values
P-values serve as a vital tool in interpreting the significance of data within various fields. Their ability to inform researchers about the strength of their findings helps in making data-driven decisions. In this section, we explore three primary areas where p-values hold substantial importance: medical research, social sciences, and business and marketing analytics. Understanding these applications provides insight into not only their practical use but also their potential impacts on research and decision-making processes.
Medical Research
In the realm of medical research, the application of p-values is incredibly significant. Researchers often employ them to determine the efficacy of new treatments or drugs. When a study tests the hypothesis that a new medication is more effective than a placebo, the p-value indicates the likelihood that observed differences are due to chance.
A smaller p-value, typically below 0.05, suggests strong evidence against the null hypothesis, meaning the treatment likely has a real effect. For instance, in clinical trials for cancer treatments, a p-value less than 0.01 may be deemed compelling enough to move forward with regulatory review. This use of p-values not only guides therapeutic decisions but shapes regulatory policies and guides funding allocation in research.
However, caution is needed because relying solely on p-values can lead to misleading interpretations. For example, an overwhelming focus on statistical significance can overshadow practical relevance or the clinical importance of findings. That’s why it’s imperative that researchers also consider effect size, which complements p-values and provides a fuller picture of the clinical implications.
Social Sciences
In the social sciences, p-values play a critical role in hypothesis testing across studies involving human behavior, societal trends, and psychological phenomena. Think of a researcher examining whether a new educational method improves student performance. The p-value helps determine if differences in exam scores are statistical noise or evidence of a genuine effect.
Social scientists often grapple with complexities that introduce variability. Cultural factors, environmental influences, and individual differences can all muddy the waters. Therefore, a p-value helps anchor findings within a broader context. If a p-value is low, it could lead to new policies or initiatives that might revolutionize educational practices or public programs.
However, it's crucial to contextualize p-values within the study design and sample size. A small sample may yield an impressive p-value, but that doesn’t mean the results are robust or generalizable to larger populations. Researchers must ensure their statistical rigor is up to snuff—otherwise, they risk riding the rollercoaster of miscommunication.
Business and Marketing Analytics
In the world of business, p-values inform data-driven strategies, particularly in marketing analytics. Companies routinely test marketing campaigns to ascertain effectiveness. Imagine a scenario where a retail brand experiments with different email promotions: by calculating p-values, they can identify whether a particular strategy outperforms others significantly.
When running A/B tests, the p-value functions as a gatekeeper for business decisions. If the p-value is consistently below the threshold of significance, it may justify scaling the successful initiative. Conversely, high p-values could signal a need for reevaluation.
That said, businesses should tread carefully. Lower p-values do not always translate into higher profit margins or customer satisfaction. It’s crucial to recognize their limitations. Factors such as market conditions, customer preferences, and timing must be evaluated alongside p-values to paint a complete picture.
Ending
In summary, p-values are not just numbers; they are critical indicators that guide decision-making across myriad fields. From creating new medical interventions to shaping social policies and driving business strategies, understanding the applications of p-values is essential. However, a nuanced approach towards interpreting these values can significantly enhance their effectiveness in research and practice. As the old saying goes, "don’t put all your eggs in one basket"—it’s prudent to use p-values in conjunction with other statistical measures for a well-rounded analysis.
Common Misconceptions about P-Values
Understanding the nuances of p-values is crucial for anyone engaging in statistical analysis. Misconceptions surrounding this concept can lead to misinterpretations, potentially skewing research results and influencing decisions in various fields. This section sheds light on some common misunderstandings that often arise in discussions about p-values. By addressing these misconceptions, we aim to foster a clearer comprehension of statistical significance and its implications.
P-Value as Proof
One of the most pervasive myths is that a p-value serves as definitive proof of a hypothesis. Many people assume that a low p-value confirms the truth of the null hypothesis or suggests that an observed effect is substantial. However, this is far from the reality. A p-value does not imply that a hypothesis is correct; instead, it indicates the likelihood of observing the data, or something more extreme, if the null hypothesis is true.
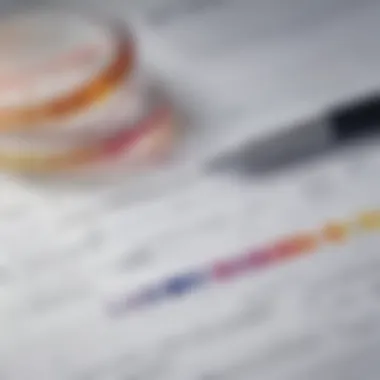
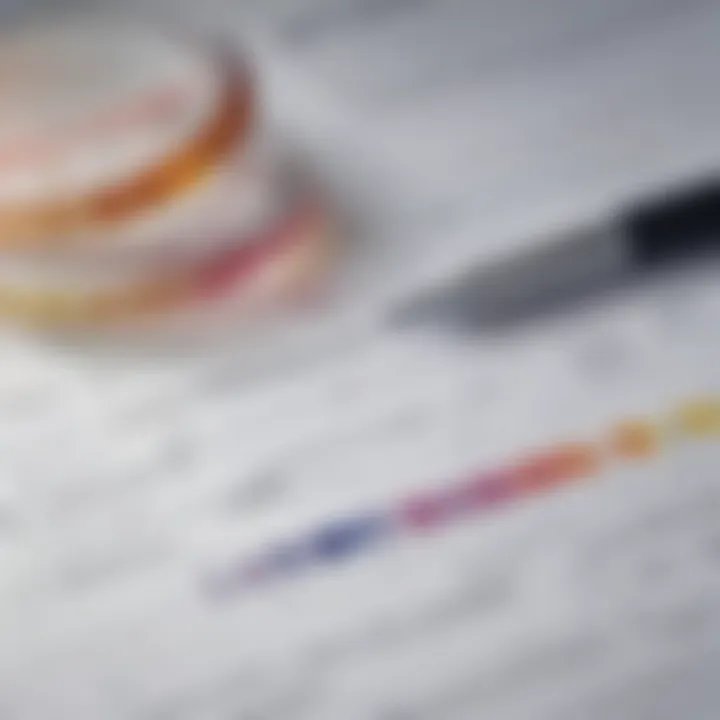
For example, consider a medical study testing a new drug. A p-value of 0.03 might suggest that there’s only a 3% probability that the observed results arose by chance, assuming the null hypothesis is true. While this suggests statistical significance, it does not endorse the efficacy of the drug; causation cannot be established through p-values alone. Thus, sticking to rigorous experimental design and considering other aspects, like the context of the study and further validation, is essential for drawing more reliable conclusions.
P-Value and Effect Size
Another significant misconception relates to the relationship between p-values and effect size. Often, researchers focus solely on whether a p-value crosses a certain threshold, like 0.05, neglecting the actual size of the effect being measured. A statistically significant p-value does not necessarily mean the effect is meaningful or large enough to be practically significant.
For instance, in a clinical trial, a new medication may show a statistically significant p-value while only delivering a minuscule increase in your cholesterol levels. This raises the question: how relevant is that effect in a real-world context? To better assess the relevance of results, one must consider both the p-value and measures of effect size, such as Cohen's d or Odds Ratios. This combined approach enables a more comprehensive understanding of findings and aids in making more informed decisions based on statistical analyses.
P-Value Thresholds
Many individuals cling to rigid p-value thresholds—typically 0.05 or 0.01—believing these numeric values are universally applicable benchmarks for significance. While these thresholds serve as helpful guidelines, they cannot capture the complexity of every research scenario. Context matters; different fields or disciplines may warrant varying levels of statistical rigor.
Moreover, the notion that p-values must be strictly below a certain threshold to hold merit introduces a binary understanding of data. In practical terms, a p-value of 0.051 will often be deemed inadequate, while 0.049 gets hailed as significant. This arbitrary division oversimplifies statistical reasoning. It’s more productive to interpret p-values in the wider context of the study, considering sample sizes and research designs, rather than as hard and fast rules.
"P-values are just one piece of the puzzle; use them as a guide, but not the gospel." – Unknown
Culmination
Alternatives to P-Values
In the realm of statistics, the p-value has long held the spotlight as a key player in hypothesis testing, but it’s not the only option. As research methods and statistical analysis have evolved, so too have the alternatives that offer different perspectives and insights. Exploring these alternatives to p-values is crucial for a more nuanced understanding of statistical evidence.
Confidence Intervals
Confidence intervals provide a broader view of data by estimating a range within which a population parameter likely falls. Instead of giving a single threshold as p-values do, confidence intervals, typically set at 95% or 99%, reflect the uncertainty around an estimate. For instance, if you’re analyzing the average return rate of an investment and establish a 95% confidence interval ranging from 5% to 10%, you can infer that you are 95% confident that the true average lies within this range. This approach encourages researchers and analysts to think about the precision of their estimates, rather than solely focusing on whether they meet a particular significance level.
- Benefits of Confidence Intervals:
- They offer more information than a mere p-value.
- The range detail helps in decision-making.
- Can show the magnitude of the effect, unlike p-values.
"A confidence interval does more than confirm findings; it illustrates the reliability and the variability inherent in the data."
Bayesian Methods
Bayesian statistics represent a shift in paradigm, relying on prior beliefs and evidence rather than solely on observed data. By integrating prior distributions with observed data, Bayesian methods update beliefs about hypotheses. This approach allows statisticians and investors alike to incorporate existing knowledge into their analyses, resulting in more tailored insights. For instance, if you are evaluating a new stock investment, you might start with historical performance as your prior and then adjust your assessments as you collect new data, yielding a more holistic view of potential risks.
- Considerations in Bayesian Analysis:
- Requires careful consideration of prior beliefs, which can be subjective.
- Often more computationally intensive than traditional methods.
- Provides a direct probability of hypotheses, unlike p-values.
Effect Size Measures
Effect size measures add another layer of depth to statistical analysis by focusing on the magnitude of an observed effect. While p-values can indicate whether an effect exists, they don’t address how substantial that effect is. Measures like Cohen's d or Pearson's r quantify the size of the difference or relationship, providing insight into practical significance. For example, a p-value may indicate a statistically significant difference between two drug treatments, but without effect size, you would miss out on understanding whether that difference is clinically meaningful.
- Benefits of Effect Size Measures:
- Helps in assessing the real-world relevance of findings.
- Offers a more comprehensive picture of results.
- Supports comparisons across studies and contexts.
Understanding these alternatives to p-values not only broadens the toolbox available for data analysis but also fosters a more critical and informed approach to interpreting results. While p-values may still hold value, employing complementary methods like confidence intervals, Bayesian approaches, and effect size measures can offer richer insights, particularly in fields where decisions hinge on statistical reasoning.
Implications of P-Value Misinterpretation
The significance of accurately interpreting p-values cannot be understated. Misinterpretation can lead to false conclusions in research, affecting industries ranging from healthcare to economics. For those navigating the complex world of statistics, understanding what a p-value represents is crucial. It's not just a number; it’s a key that can open doors to meaningful insights or lead individuals down the wrong path if not handled carefully.
False Discoveries
One of the most alarming consequences of misinterpreting p-values is the phenomenon of false discoveries. This occurs when a p-value is mistakenly viewed as strong evidence for a hypothesis when, in fact, it isn't. A common error is setting a p-value threshold of 0.05 and believing any result below this is significant without further scrutiny. For example, if a study claims to find a link between a new medication and improved health outcomes, a falsely significant p-value could give a false sense of security.
"A p-value does not tell us the probability that the null hypothesis is true. It merely informs us about the data under the assumption that the null hypothesis holds true."
The implications can be dire. In medical research, this might lead to the adoption of ineffective treatments, wasting resources and potentially endangering lives. Similarly, in social sciences, policies based on flawed data may reinforce stereotypes or lead to unjust amplifications of certain social issues.
Reproducibility Crisis
The misinterpretation of p-values contributes significantly to what many researchers are calling the reproducibility crisis. This crisis refers to the increasing difficulty in replicating study results. Many findings with initially low p-values are unable to hold up under further testing. When researchers publish studies based solely on statistically significant p-values, they may overlook the true variability in data.
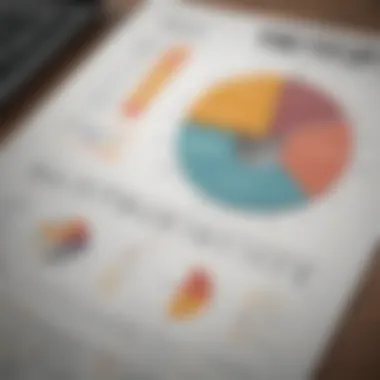
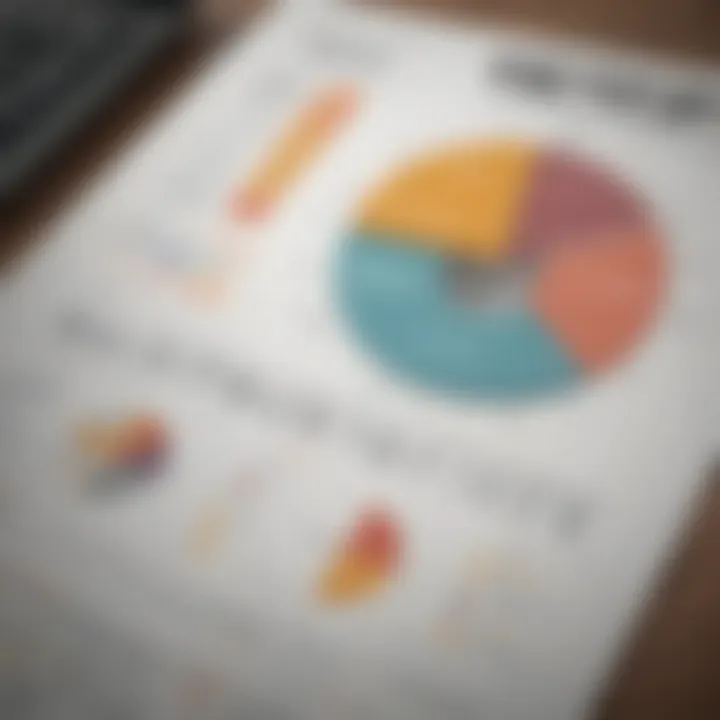
As a result, the foundation of many scientific disciplines becomes shaky. When subsequent studies fail to reproduce findings, it leads to distrust in scientific methods and can slow down the pace of discovery. Educational efforts aimed at improving statistical literacy among researchers can mitigate this issue. It's important to teach not just how to calculate p-values but also how to interpret them in the larger context of research.
Ethical Considerations
Lastly, there are pivotal ethical considerations tied to the interpretation of p-values. When researchers or analysts misinterpret these values, they risk contributing to misinformation. This is particularly problematic in industries like finance, where investment decisions based on flawed data can have significant economic repercussions. Educators, students, analysts, and investors alike must recognize their responsibility in using p-values correctly.
The stakes become even higher when public health policies are influenced by such misinterpretation. A skewed understanding of what a p-value signifies can lead to policies that harm rather than promote societal well-being. Therefore, integrity in data reporting and interpretation isn't just a best practice; it’s an ethical obligation.
In summary, the implications of p-value misinterpretation resonate deeply across various fields. From triggering false discoveries to contributing to the reproducibility crisis and raising ethical questions, the responsibility lies heavily on the shoulders of researchers to uphold a high standard of accuracy and transparency in their work.
Best Practices for Reporting P-Values
When it comes to reporting p-values, adherence to best practices can greatly impact the quality and clarity of statistical findings. This section focuses on the critical elements that ensure accurate interpretation and presentation of p-values, which is essential for fostering trust and understanding among the audience—be it researchers, stakeholders, or the general public. Proper reporting helps mitigate misinterpretations, thereby enhancing the communication of scientific results.
Transparency in Reporting
One of the cornerstones of statistical reporting is transparency. Being candid about methodologies, assumptions, and limitations surrounding p-value calculations adds credibility to the findings. This can involve including details such as:
- The sample size used in the analysis
- The specific statistical tests undertaken
- Any adjustments made for multiple testing
- The context in which the data was collected
By laying these cards on the table, researchers allow for more informed scrutiny and reproducibility of results. Ultimately, transparency paves the way for a healthier academic dialogue. After all, it's like showing your work in math class; it helps others follow your thought process.
Reporting Complete Results
Simply providing a p-value without context can lead to confusion. Best practices dictate that researchers should report not just the p-value but also the relevant effect sizes and confidence intervals where applicable.
Consider the following elements when reporting results:
- P-Value: This indicates the probability of observing the data assuming the null hypothesis is true.
- Effect Size: This provides insight into the magnitude of the effect, going beyond mere statistical significance.
- Confidence Intervals: By reporting confidence intervals, you offer a range in which the true effect size likely lies, helping to paint a fuller picture.
A well-rounded report gives a clearer understanding of the implications of the findings. Instead of stating "p 0.05," one could elaborate, "The p-value is 0.03, suggesting a statistically significant result, alongside a medium effect size of 0.4, with a 95% confidence interval ranging from 0.2 to 0.6."
Contextualizing Findings
Context is everything. Providing a backdrop for the reported results is vital for proper interpretation. This means discussing:
- Theoretical implications: How does the p-value fit into existing research or theories within the field?
- Practical significance: What does the result mean in real-world terms, especially for decision-makers in industries like healthcare, business, or education?
- Limitations: Acknowledging shortcomings helps manage expectations and suggests areas for future research.
For example, a researcher examining a new drug might find a p-value of 0.01. However, without knowing the clinical relevance or the study's limitations, stakeholders might interpret this finding differently. Contextualization aids in shaping a nuanced understanding that goes beyond mere numbers.
"Context acts like a lens; it transforms raw data into valuable insights."
Reporting p-values is not just a matter of stating numbers; it's about presenting a narrative that conveys meaning, relevance, and insight. By following best practices in transparency, completeness, and contextualization, researchers can effectively communicate their findings and contribute constructively to their field.
Future Directions in P-Value Research
The conversation around p-values is as vibrant as a street market; ongoing discussions reflect the dynamic state of statistical science. While p-values have held a prominent place in statistical analysis, their limitations have sparked a quest for a more nuanced understanding of what they really convey in research. The future beckons towards a shift, with a clear need to evolve beyond traditional approaches. This section will delve into the critical components of evolving standards, new methodologies, and the education of upcoming researchers.
Evolving Statistical Standards
As we navigate through the post-meaningful p-value era, it’s paramount for statistical standards to advance. The older methods focused solely on the p-value are becoming less satisfactory. Emerging frameworks emphasize a broader context, suggesting that relying solely on p-values may lead to oversimplified conclusions. Adapting standards implies:
- Debating the Significance Level: The common alpha level of 0.05 is under scrutiny. Different fields may require tailored significance levels that reflect their unique challenges.
- Incorporating More Metrics: Statistical reports increasingly spotlight effect sizes and confidence intervals, viewing the p-value as part of a larger picture rather than as a standalone indicator.
This evolution pushes researchers to embrace a richer statistical toolkit. As researchers refine their methodologies, they also trend towards transparency; unveiling how conclusions are drawn helps build trust in scientific results.
Integration of New Methods
Integrating innovative statistical methods is vital for revitalizing p-value interpretation. Several promising methodologies are gaining momentum:
- Bayesian Statistics: Unlike traditional frequentist approaches, Bayesian analysis can incorporate prior knowledge, offering a richer interpretation of data. This method allows statisticians to update their beliefs as new data emerges.
- Machine Learning Techniques: The advent of artificial intelligence brings forth sophisticated algorithms that can discern patterns and associations without being shackled by classical p-values' limitations.
- Meta-Analysis and Network Analysis: These approaches facilitate the aggregation of findings across studies, allowing for a more encompassing view of research trends and data significance.
The future may well see p-values coexisting with these methodologies, providing a layered comprehension of statistical outcomes that better serves fields spanning health care to finance.
Educating Researchers
A conscientious effort to educate researchers on p-value implications stands at the heart of future directions in p-value research. Universities and institutions play a key role in this educational shift. It shouldn’t just be about crunching numbers but understanding narratives they tell. Education should aim at:
- Workshops and Seminars: Offering regular programs focusing on modern statistical techniques can keep practitioners in sync with evolving standards.
- Curriculum Revisions: Updating educational content to highlight the importance of complementing p-values with effect sizes, confidence intervals, and practical implications.
- Promoting Critical Thinking: Encouraging learners to question data rather than accept figures at face value is essential. Understanding the context of p-values in research design leads to deeper insight.
It's not about rejecting p-values. Rather, it's about recognizing their place in a more complex statistical narrative.
Amidst the changing landscape, the guiding principle should be clear communication of results, emphasizing that statistics is inherently a disciplined art requiring both rigor and creativity.